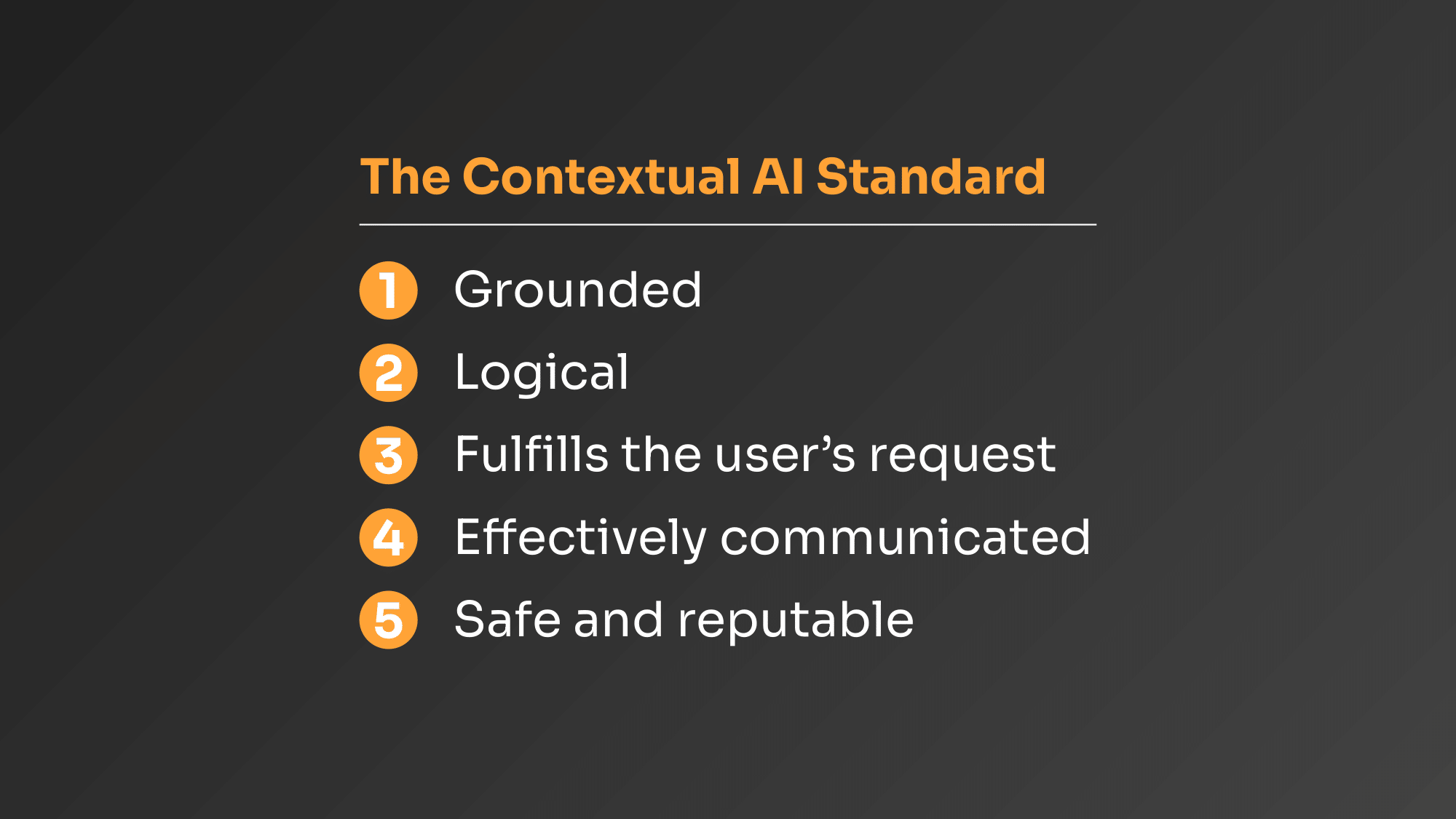
Building better agents: The critical role of human-annotated data
Enterprises are rapidly adopting AI agents to empower their knowledge workers, and the next several years will see a huge productivity boom. By using agents to become more effective and efficient, skilled teams will increasingly be freed up to focus on higher value and uniquely human tasks.
At Contextual AI, we are helping some of the most innovative companies bring AI into production with our platform for building specialized RAG agents, solving frontier AI problems that off-the-shelf solutions cannot address. Our approach enables customers to deploy AI that’s precisely tailored to their unique business contexts and knowledge.
Labelled data is a core part of how we specialize agents to meet customer requirements and evaluate them against production deployment criteria. High-quality data has helped us solve unique challenges that each enterprise customer’s use case presents. Without it, we would not be able to customize agents for complex domains like finance, semiconductors, and professional services.
In this post, I’ll first explain some of the key components of our Data team’s strategy for generating human-annotated data. Then, I’ll introduce the Contextual AI Standard—our team’s framework for defining what good labelled data looks like and, by extension, how we want specialized RAG agents to behave.
How our data engine empowers customers and internal teams
The Contextual AI Data team’s mission is to make sure that our customers and internal teams have the right data. The data we provide enables our customers and our Customer ML Engineering team to specialize and evaluate agents for specific use cases. The data also enables our Research and Product teams to build capabilities for real-world enterprise use cases. While we use open-source industry benchmarks to gauge our performance (see our latest state-of-the-art results), we get far better signals from customer-specific evaluation datasets that ensure we are improving real customer outcomes rather than chasing vanity numbers.
Putting this into practice, the Data team is responsible for creating and managing high-quality labelled datasets that faithfully represent real customer use cases. This means:
- The right quality of data: it has to be realistic, diverse, challenging, and labelled consistently using the right categories
- The right quantity of data: as they say, quantity has a quality all of its own
- Data delivered at the right time: it has to be timely—great data a week late has no use in a fast-moving startup
Unlike most AI companies, we have an in-house team of annotators who are often tasked with turning around data deliveries in just 24 hours. To make this work, we have our own labeling frameworks and bespoke guidance, maintain a tight quality control and feedback system, and are constantly innovating to improve the quality-time tradeoff. We do occasionally work with outside vendors, but only ones we trust and for targeted projects.
Sometimes customers give us labelled datasets with example queries and ideal responses. However, a core part of the Data team’s work is creating datasets entirely from scratch to specialize and evaluate agents. This is a tricky process—annotators have to first put themselves in the shoes of our customers and come up with a realistic input query. They then have to hop over the fence and create a high-quality, gold-standard response. Because we prioritize explainability and auditability, the annotators also have to record all the steps they took to evidence their responses, such as searching through documents, reasoning about them, and calling APIs/tools. Only experts in each domain (e.g., finance, hardware, digital technology) can do this well.
Defining success with the Contextual AI Standard
Tasking annotators with creating datasets from scratch raises an obvious question: What does a gold-standard response from a specialized RAG agent look like?
The easy answer is “it depends”—different customers, industries, functions, and use cases all have nuances that create different success criteria. However, because of our focus on enterprise use cases, there is a fairly clear boundary around the universe of possible good responses—we have not yet spoken with a customer who wants an agent that hallucinates and makes things up!
With this in mind, over the past year, we’ve developed the Contextual AI Standard. It comprises clear criteria for high-quality model responses. We use these criteria to evaluate our agents’ responses, creating consistent and easily understood requirements that help us systematically diagnose performance gaps and address those gaps.
- Grounded – All factual claims in the response are entailed by the evidence.
- Logical – All analyses and calculations in the response should be logical and tied back to the factual claims.
- Fulfills the user’s request – The response should directly and completely address the user’s query (i.e., it should be helpful and useful).
- Effectively communicated – The response should be engaging and build trust with the user, and it should meet their specific expectations for style.
- Safe and reputable – The response should not be harmful, biased, reputationally damaging or unethical.
Each criterion is associated with numerous rules that make the fairly abstract requirements more tangible. Each can be judged by annotators or used as goals to train our AI systems. Operationally, the Contextual AI Standard has massively improved agreement amongst annotators and helped save a lot of time. We are now converting parts of the Standard into AI-based quality control tools, powered by our own platform, to help drive more efficiency within the team.
Some of our partners have asked whether the Standard is too restrictive because it involves prescribing a clear view on quality. This is a good point, and it’s important to see the Standard as a living document, with every requirement constantly being scrutinized as we refine our platform vision. The components are modular, which means we can adjust them based on customers’ requirements—one rule might not be a good fit for a certain use case.
Our operational experience with using the Contextual AI Standard points to a more fundamental truth—being prescriptive, paradoxically, makes it easier to be flexible. Once you have a framework and shared language in place, you can diverge from it.
Conclusion
At Contextual AI, we have taken a big strategic bet on data, investing in it to help us innovate and improve the capabilities and performance of the Contextual AI Platform.
Our in-house annotation team and the Contextual AI Standard are just two of the strategic choices we’ve made, and we aim to share more about our approach throughout 2025.
If you want to find out more, reach out to data@contextual.ai.